GO-VMP: Global Optimization for View Motion Planning in Fruit Mapping
Authors:
A. Isaac Jose, S. Pan, T. Zaenker, R. Menon, S. Houben, M. BennewitzType:
PreprintPublished in:
Arxiv Pre-printYear:
2025Related Projects:
AID4Crops - Automation and AI for Monitoring and Decision Making of Horticultural Crops, Phenorob - Robotics and Phenotyping for Sustainable Crop ProductionLinks:
BibTex String
@article{isaacjose2025govmp,
title={{GO-VMP}: {G}lobal Optimization for View Motion Planning in Fruit Mapping},
author={Isaac Jose, Allen and Pan, Sicong and Zaenker, Tobias and Menon, Rohit and Houben, Sebastian and Bennewitz, Maren},
journal={arXiv preprint arXiv:2503.03912},
year={2025}
}
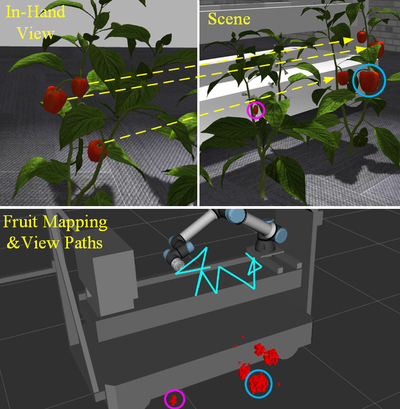
Abstract:
Automating labor-intensive tasks such as crop monitoring with robots is essential for enhancing production and conserving resources. However, autonomously monitoring horticulture crops remains challenging due to their complex structures, which often result in fruit occlusions. Existing view planning methods attempt to reduce occlusions but either struggle to achieve adequate coverage or incur high robot motion costs. We introduce a global optimization approach for view motion planning that aims to minimize robot motion costs while maximizing fruit coverage. To this end, we leverage coverage constraints derived from the set covering problem (SCP) within a shortest Hamiltonian path problem (SHPP) formulation. While both SCP and SHPP are well-established, their tailored integration enables a unified framework that computes a global view path with minimized motion while ensuring full coverage of selected targets. Given the NP-hard nature of the problem, we employ a region-prior-based selection of coverage targets and a sparse graph structure to achieve effective optimization outcomes within a limited time. Experiments in simulation demonstrate that our method detects more fruits, enhances surface coverage, and achieves higher volume accuracy than the motion-efficient baseline with a moderate increase in motion cost, while significantly reducing motion costs compared to the coverage-focused baseline. Real-world experiments further confirm the practical applicability of our approach.