Constrained Object Placement Using Reinforcement Learning
Authors:
B. Kreis, N. Dengler, J. de Heuvel, R. Menon, H. D. Perur, M. BennewitzType:
PreprintPublished in:
arXiv preprintYear:
2024Related Projects:
RePAIRDOI:
https://doi.org/10.48550/arXiv.2404.10632Links:
BibTex String
@article{kreis2024constrained,
title={Constrained Object Placement Using Reinforcement Learning},
author={Benedikt Kreis and Nils Dengler and Jorge de Heuvel and Rohit Menon and Hamsa Datta Perur and Maren Bennewitz},
journal={arXiv preprint arXiv:2404.10632},
year={2024},
doi={10.48550/arXiv.2404.10632}
}
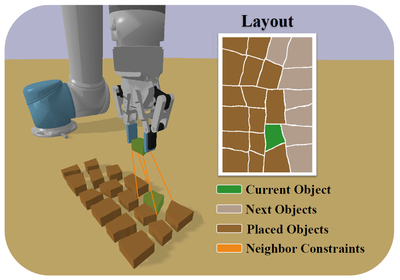
Abstract:
Close and precise placement of irregularly shaped objects requires a skilled robotic system. Particularly challenging is the manipulation of objects that have sensitive top surfaces and a fixed set of neighbors. To avoid damaging the surface, they have to be grasped from the side, and during placement, their neighbor relations have to be maintained. In this work, we train a reinforcement learning agent that generates smooth end-effector motions to place objects as close as possible next to each other. During the placement, our agent considers neighbor constraints defined in a given layout of the objects while trying to avoid collisions. Our approach learns to place compact object assemblies without the need for predefined spacing between objects as required by traditional methods. We thoroughly evaluated our approach using a two-finger gripper mounted to a robotic arm with six degrees of freedom. The results show that our agent outperforms two baseline approaches in terms of object assembly compactness, thereby reducing the needed space to place the objects according to the given neighbor constraints. On average, our approach reduces the distances between all placed objects by at least 60%, with fewer collisions at the same compactness compared to both baselines.