Subgoal-Driven Navigation in Dynamic Environments Using Attention-Based Deep Reinforcement Learning
Authors:
J. de Heuvel, W. Shi, X. Zeng, M. BennewitzType:
Conference ProceedingPublished in:
IEEE International Conference on Advanced Robotics (ICAR)Year:
2023Related Projects:
FOR 2535 - Anticipating Human BehaviorLinks:
BibTex String
@INPROCEEDINGS{deheuvel_23_subgoal,
author={De Heuvel, Jorge and Shi, Weixian and Zeng, Xiangyu and Bennewitz, Maren},
booktitle={2023 21st International Conference on Advanced Robotics (ICAR)},
title={Subgoal-Driven Navigation in Dynamic Environments Using Attention-Based Deep Reinforcement Learning},
year={2023},
volume={},
number={},
pages={79-85},
keywords={Laser radar;Navigation;Velocity control;Computer architecture;Reinforcement learning;Robot sensing systems;Collision avoidance},
doi={10.1109/ICAR58858.2023.10406349}
}
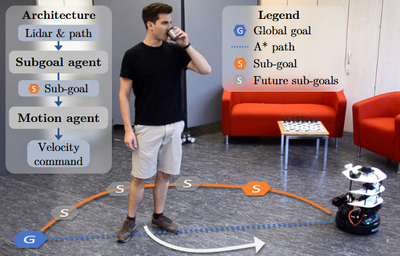
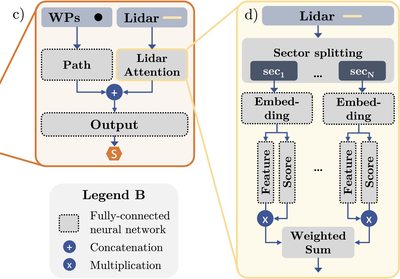
Abstract:
Collision-free, goal-directed navigation in environments containing unknown static and dynamic obstacles is still a great challenge, especially when manual tuning of navigation policies or costly motion prediction needs to be avoided. In this paper, we therefore propose a subgoal-driven hierarchical navigation architecture that is trained with deep reinforcement learning and decouples obstacle avoidance and motor control. In particular, we separate the navigation task into the prediction of the next subgoal position for avoiding collisions while moving toward the final target position, and the prediction of the robot's velocity controls. By relying on 2D lidar, our method learns to avoid obstacles while still achieving goal-directed behavior as well as to generate low-level velocity control commands to reach the subgoals. In our architecture, we apply the attention mechanism on the robot's 2D lidar readings and compute the importance of lidar scan segments for avoiding collisions. As we show in simulated and real-world experiments with a Turtlebot robot, our proposed method leads to smooth and safe trajectories among humans and significantly outperforms a state-of-the-art approach in terms of success rate. A supplemental video describing our approach is available online.