Predicting Human Navigation Goals based on Bayesian Inference and Activity Regions.
Authors:
L . Bruckschen, K. Bungert, N. Dengler, M. BennewitzType:
ArticlePublished in:
Robotics and Autonomous Systems (RAS)Year:
2020DOI:
https://doi.org/10.1016/j.robot.2020.103664.Links:
BibTex String
@article{bruckschen20ras,
AUTHOR = {L. Bruckschen and K. Bungert and N. Dengler and M. Bennewitz},
TITLE = {Predicting Human Navigation Goals based on {B}ayesian Inference and Activity Regions},
YEAR = 2020,
JOURNAL = {Robotics and Autonomous Systems (RAS)}
}
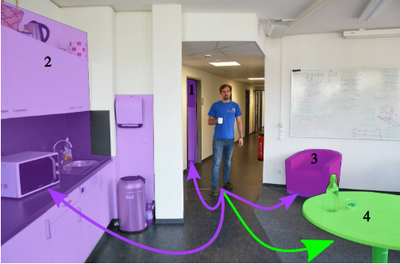
Abstract:
Anticipation of human movements is of great importance for service robots, as it is necessary to avoid interferences and predict areas where human-robot collaboration may be needed. In indoor scenarios, human movements often depend on objects with which they interacted before. For example, if a human interacts with a cup the probability that a table or coffee machine might be the next navigation goal is high. Typically, objects are grouped together in regions depending on the related activities so that environments consist of a set of activity regions. For example, a workspace region may contain a PC, a chair, and a table with many smaller objects on top of it. In this article, we present an approach to predict the navigation goal of a moving human in indoor environments. We hereby combine prior knowledge about typical human transitions between activity regions with robot observations about the human’s current pose and the last object interaction to predict the navigation goal using Bayesian inference. In the experimental evaluation in several simulated environments we demonstrate that our approach leads to a significantly more accurate prediction of the navigation goal in comparison to previous work. Furthermore, we show in a real-world experiment how such human motion anticipation can be used to realize foresighted navigation with an assistance robot, i.e. how predicted human movements can be used to increase the time efficiency of the robot’s navigation policy by early anticipating the user’s navigation